


CLINICAL IMPLEMENTATION of COMPUTATIONAL PATHOLOGY
The main goal of this research is to leverage the expertise of subspecialty trained academic pathologists to pursue tissue-based biomarker research on prostate, kidney and bladder cancer. We analyze mechanisms in human tissues using Multi-OMIC data collections from local cohorts to investigate cancer evolution, tumor heterogeneity and progression to metastatic disease.
We also conduct research to understand how pathologists evaluate and utilize software solutions in digital pathology that can potentially assist their sign-out of cases. These research projects include a commercial algorithm and user interface for diagnosis and grading of prostate cancer and an in-house developed workflow for quantification of PD-L1 IHC in lung cancer.
Meet our Researchers
Multi-OMIC investigation
Research Includes:
* Tissue microarrays capture tumor heterogeneity
* Parallel multiplex immunofluorescence, IHC and RNA & DNA sequencing data
* Spatial profiling of cancer associated pathways and cell phenotypes
* Novel machine learning algorithms to detect seminal vesicle invasion and extracapsular extension
* Radiology – pathology integration
* Clinical outcomes studies
Multiplex tissue staining with sequential immunofluorescence (IF) and chromogenic detection (IHC) of protein or RNA;

An Independent Prospective Validation of a Deep Learning Algorithm for Prostate Cancer Detection and Gleason Grading
Presentation at USCAP 112th Annual Meeting: Facing the Unknown.
Daniel Albertson1, In Hye Suh2, Sheila Mota1, Marc Barry1, Jonathon Mahlow1, Beatrice S. Knudsen1, Deepika Sirohi1, Tae-Yeong Kwak2, Yoon Min Lee2, Sun Woo Kim2, Hyeyoon Chang1
Affiliations: 1 Department of Pathology, University of Utah, USA; 2 Deep Bio Inc., Seoul, South Korea
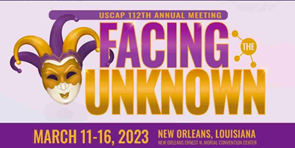
The study includes:
* 1701 prostate biopsy slides from 141 consecutive patients
* A reference standard based on a consensus of four GU subspecialty pathologists was constructed from 599 slides of 101 patients.
* Compared to the reference standard, the DeepDx® Prostate possessed a sensitivity of 0.992 and a specificity of 0.844 for cancer detection.
* The quadratic-weighted Cohen’s kappa for assigning the Gleason grade group by DeepDx® Prostate was 0.820.
Conclusion: This study validates the AI algorithm in an independent set of cases that the algorithm had not previously seen and also highlights its potential benefit as a high-sensitivity tool in anatomic pathology practice.

A pipeline for evaluation of machine learning/AI models to quantify PD-L1 immunohistochemistry
Key Results:
* Need for cancer segmentation to improve PD-L1 tumor proportion scores
* Less than 20 cases are required to adapt a model to local cases using a transfer learning approach
* Training on negative control and PD-L1 stained slides improves the performance of the model
* A systematic digital pathology pipeline provides a framework that helps pathologists gain trust in computer-aided scoring of PD-L1

Histologic Growth Patterns (HGP) in Clear Cell Kidney Cancer
Histologic Growth Patterns in Clear Cell Renal Cell Carcinoma Stratify Patients into Survival Risk Groups.
Sirohi D, Chipman J, Barry M, Albertson D, Mahlow J, Liu T, Raps E, Haaland B, Sayegh N, Li H, Rathi N, Sharma P, Agarwal N, Knudsen B.
Clin Genitourin Cancer. 2022
We demonstrated that HGPs in kidney cancer predict the risk of cancer recurrence as well as ISUP/WHO nuclear grades. Our research is identifying hidden genomic and morphologic features of HGPs and vascular organization within HGPs that are associated with disease progression and response to therapy.
